Introduction
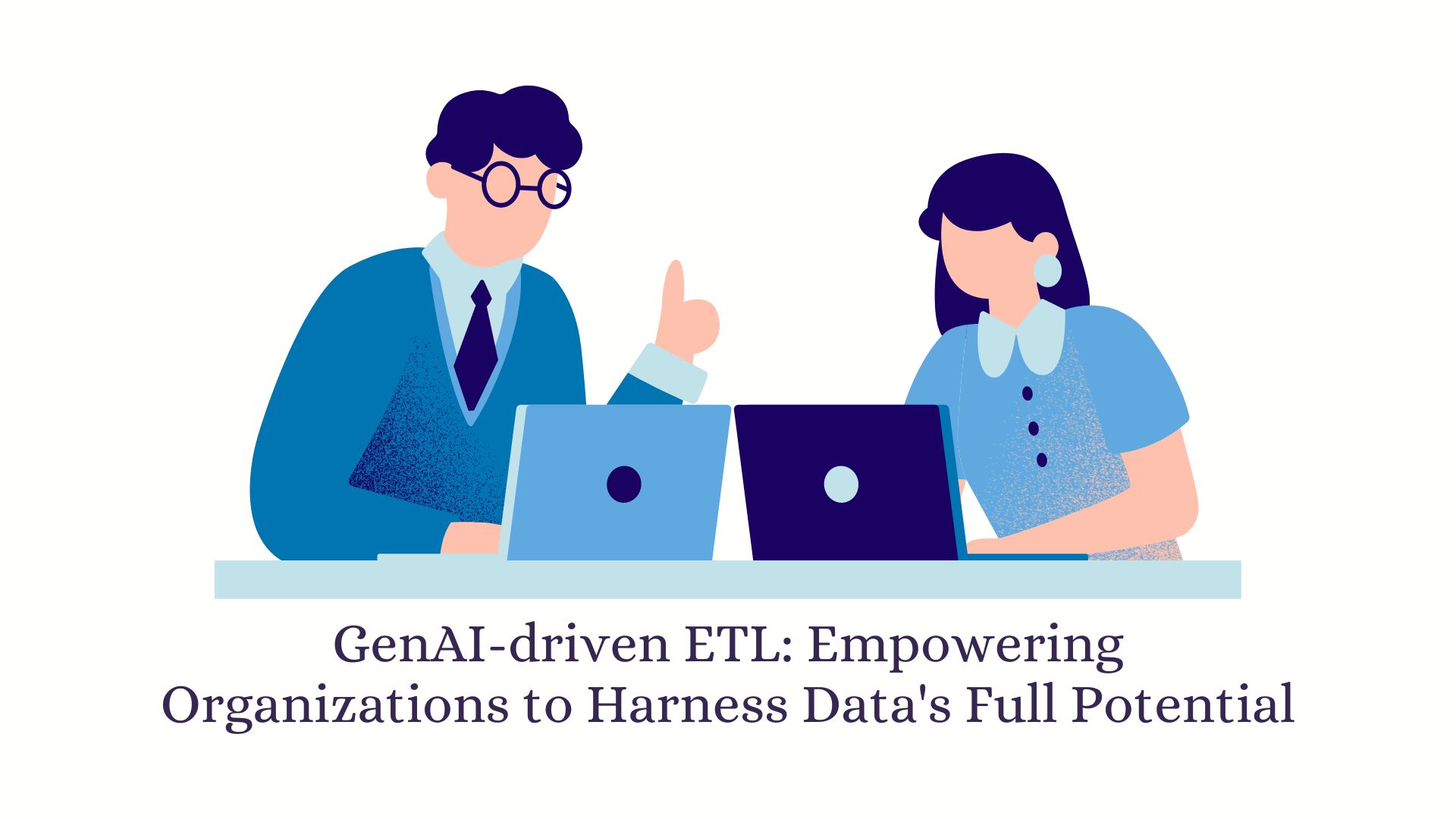
In today’s digital world, Data is like oil, fueling businesses and guiding decisions. However, large amounts of Data are difficult to handle efficiently. Traditional techniques like ETL struggle to keep up. Enter GenAI-driven ETL – a game-changer in the Data Analytics world. Using smart algorithms and automation, GenAI simplifies Data processing, saving organizations time and money.
This blog post dives into how GenAI-driven ETL is transforming Data Engineering, making it easier for organizations to better manage their Data.
The Evolution of Data Engineering:
To demonstrate the importance of GenAI-driven ETL in Data Engineering transformation, it is important to understand the challenges posed by traditional ETL systems. Traditionally, ETL involves extracting Data from sources, converting it to a suitable format, and loading it into a target database or Data Warehouse. Although this approach has served organizations well for many years, it has limitations.
First, traditional ETL processes are often development-driven and time-consuming, requiring considerable manual effort to extract, store, and manipulate Data. Furthermore, as the volume of Data increases, traditional ETL tools are very extensive and struggle to develop and create operational challenges and resource constraints.
Furthermore, the rigid nature of traditional ETL pipelines makes them less flexible in terms of the performance of evolving needs and Data Sources, resulting in inefficiencies and lost opportunities.
Enter GenAI: Transforming Data Engineering Landscape
GenAI represents a seismic shift in Data technology, providing a more dynamic and intelligent view of ETL processes. At its core, GenAI harnesses the power of artificial intelligence and machine learning to automate and optimize every aspect of the Data Pipeline. Let’s explore how GenAI-driven ETL changes the ETL process step by step:
Data Extraction
Traditional ETL systems often struggle to extract Data from a variety of unstructured sources, including social media feeds, IoT devices, and multimedia content. GenAI-driven ETL addresses this challenge to analyze and extract information from a wide variety of sources using advanced natural language processing (NLP), computer vision, and deep learning By automatically visualizing Data with the right plans and procedures, GenAI accelerates the data extraction process.
Data Modification
Data transformation is an important but software developer-driven part of the ETL process, including Data cleansing, enrichment, and aggregation. GenAI-driven ETL simplifies this process by using machine learning algorithms to automate repetitive tasks and intelligently account for Data transformations.
Through techniques such as predictive modelling and clustering, GenAI-driven ETL can identify and correct inaccurate Data, enrich datasets with context, and aggregate Data for Analysis. This not only reduces the time and effort required for Data transformation but it increases the quality and accuracy of the resulting datasets.
Data Processing
Once the Data has been extracted and transformed, it must be stored in a targeted database or Data Warehouse for analysis and reporting purposes. Traditional ETL tools often struggle to load large amounts of Data efficiently, leading to performance bottlenecks and scalability issues.
GenAI-driven ETL addresses this challenge by optimizing Data load processes through intelligent parallelization, compression, and Data partitioning techniques. By distributing Data across multiple nodes and using a distributed computing infrastructure, GenAI ensures fast and reliable Data loads, enabling organizations to optimize their Data assets.
Transforming ETL Processes with GenAI
So, how exactly does GenAI change ETL processes? Let’s take a closer look at some key strategies:
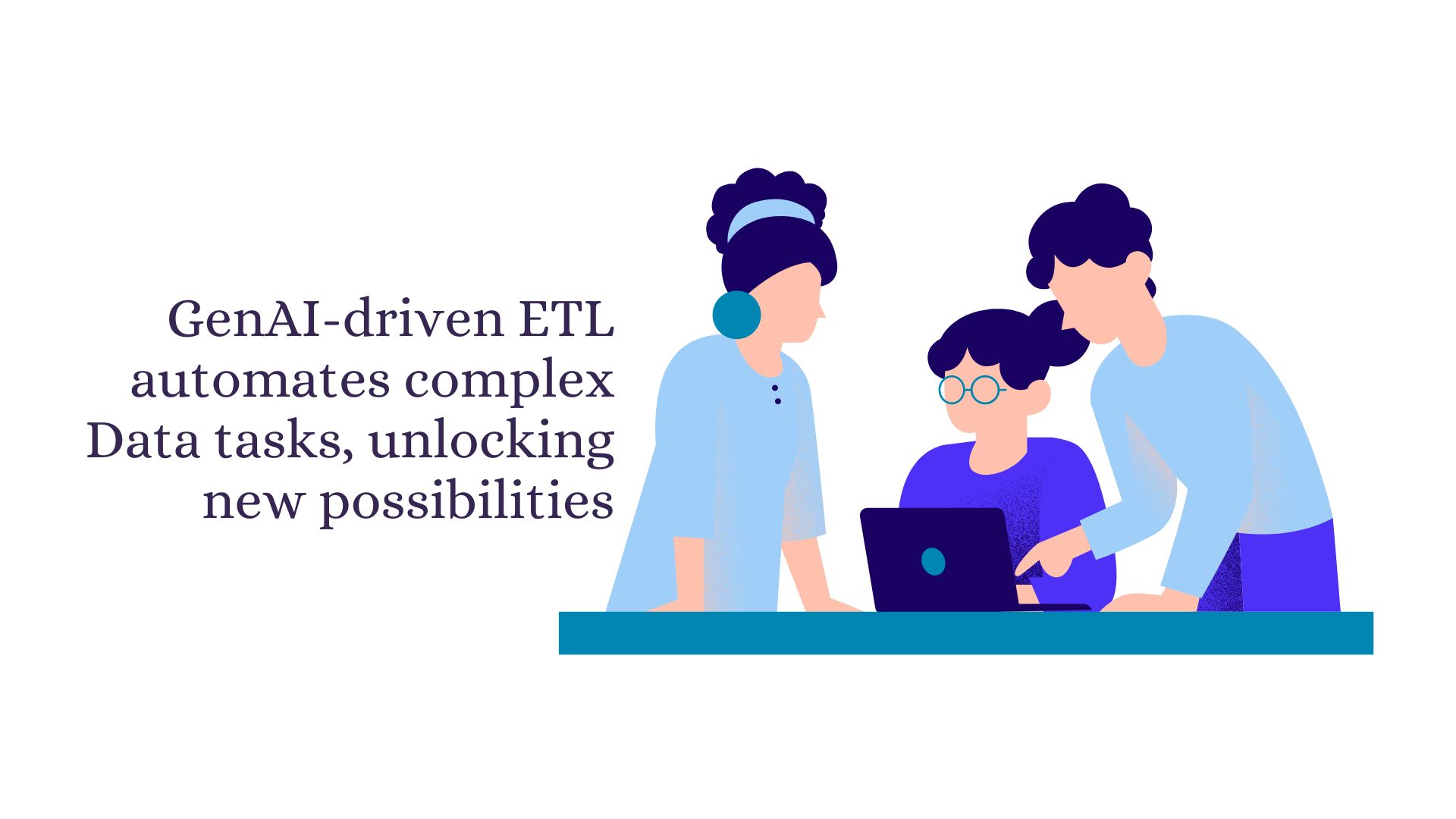
Automated Data transformation
GenAI models can learn from historical Data and automatically identify patterns and relationships in the Data. This allows complex Data transformations to be performed automatically, reducing the need for manual typing and intervention. For example, GenAI-driven ETL can automatically detect outliers, set missing values, and normalize Data, simplifying the conversion phase of ETL pipelines.
Natural Language Processing (NLP) for Data Integration
Many Data sources today are raw or semi-structured information, such as customer surveys, social media posts, or email communications GenAI-driven ETL models using natural language processing capabilities will analyze and extract context this text source can, which enables seamless integration in the ETL process This enables organizations to use multiple Data sources for analysis and decision making.
Continuous learning and adaptation
One of the key advantages of GenAI-driven ETL is its ability to continuously learn and adapt to new Data models and needs. Unlike traditional ETL systems, which often require manual modifications and rewrites as Data evolves, GenAI models are able to dynamically adjust their modifications based on Databases . This enables real-time operations and analytics, ensuring that organizations can draw insights from the latest Data.
Scalability and Performance
As the amount of Data increases exponentially, scalability becomes more important in ETL systems. GenAI-based solutions are inherently scalable, allowing organizations to manage large amounts of Data efficiently and cost-effectively. By using distributed computing infrastructure and parallel processing techniques, GenAI-driven ETL can process large Data sets quickly, significantly reducing downtime and transaction costs
Error Detection and Correction
Traditional ETL processes are prone to errors, which can lead to inconsistencies and inaccuracies in the underlying Data. GenAI-driven ETL models can help mitigate this risk by identifying and correcting errors during the transition. By learning from historical Data and identifying anomalous patterns, GenAI can predict potential errors before they are passed through the pipeline, ensuring Data quality and reliability
Conclusion
In conclusion, GenAI-driven ETL represents a paradigm shift in Data technology, delivering powerful and intelligent solutions for organizations to overcome the challenge of traditional ETL systems. Executing the power of artificial intelligence and machinery the learning in the leaves plays a role Being to unlock the full potential, driving actionable insights, informed decision making and competitive advantage in today’s Data-driven world.
As we continue to see the rapid development of AI and machine learning technologies, GenAI’s transformative impact on Data technology transforms businesses poised to do, reshape business processes and fuel innovation for years to come.